Why predictive behavioral analytics will forever change retargeting
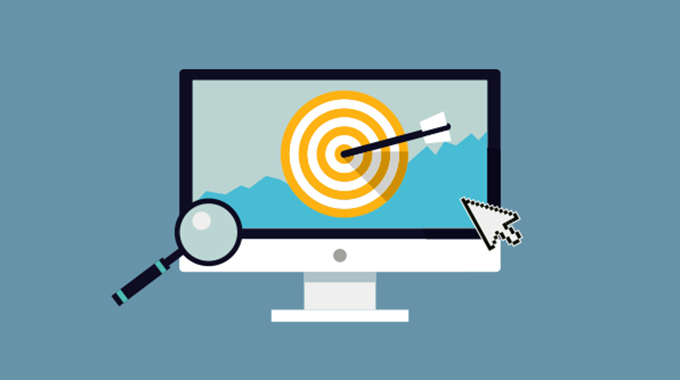
Retargeting treats all customers the same way, regardless of their on-site behavior. Here’s why behavioral targeting with algorithms is poised to upend that model.
In standard retargeting, a consumer visits an ecommerce site like Amazon and then goes about his or her day. Visiting other sites like CNN, he or she will see Amazon ads, provided Amazon outbid other interested advertisers like Best Buy or Target.
Most companies retarget consumers the same way, no matter what their intent. If they see a potential buyer who has visited their site and browsed products – or even placed products in a cart – they bid for ad space to retarget once that customer leaves.
But think about this for a moment: The customer who placed an item in a cart is much closer to making an actual purchase. Compared with the customer who simply browsed a few products, he or she is more likely to return and complete the transaction if he or she sees an ad on another site.
Let’s say a customer browsed BestBuy and then visited Target, and actually put a product in a cart. Historically, Best Buy and Target would go on to target this customer in the same manner. But it doesn’t make sense to do it like this.
The customer was much closer to buying a product from Target. As a result, Target should be advertising to this specific customer because Target has a better chance of actually selling this person something. But if Best Buy outbids Target, this customer may very well see ads for products he or she isn’t as likely to purchase. And Best Buy is frankly wasting its money.
Let’s be smart about Retargeting
I think algorithmic retargeting is the next big thing in online advertising. By developing an algorithm that segments customers based on site behavior, as well as where they are in the purchase cycle, brands can increase the effectiveness of their retargeting programs, thereby reducing the cost.
We can gauge level of interest, as well as specific areas of interest, and predict customers’ intent. Brands can then use that data to pinpoint their retargeting efforts on those customers most likely to make a purchase.
This means that instead of spending millions of dollars to blindly retarget all customers at all stages, companies can aggressively target those closest to purchase, spend ad dollars more effectively, and increase conversions.
Algorithmic/behavioral retargeting with Lenovo
Lenovo is one brand testing out algorithmic retargeting alongside classic retargeting. In the former, it is using behavioral models – which include 300 to 400 variables – based on demographic and psychographic data.
“When we really looked at the observed data, it was a small group in our case – 1 percent of customers are responsible for most purchases,” says Ajit Sivadasan, vice president and general manager of global ecommerce at Lenovo. “And the difference between those who buy and don’t is almost 900 times. People who buy are 900 times higher in terms of their per-unit transaction value, versus those who don’t have a propensity to buy.”
A better customer experience
Sivadasan also noted that with standard retargeting, brands end up giving all potential customers the same experience. Lenovo focuses on the 1 percent of people who buy, opting not to inundate the other 99 percent with marketing.
Or, as Ashish Braganza, the brand’s director of global business intelligence, put it, Lenovo was very rules-based before these tests. The rule was generally that if someone abandoned their cart, they would be retargeted all over the place.
“If they abandoned and went to, say, Yahoo or MSN or CNET, we would be retargeting because we’re buying inventory to retarget. It’s very spray-and-pray methodology,” says Braganza. “You don’t know if you should spend money on that person, [his or her] value and propensity to buy a product.”
With algorithmic retargeting, however, Lenovo can create clusters of high- and low-value customers and buy media accordingly. Beyond just the shopping carts, the brand looks at what other products someone has viewed and engaged with.
Analyzing what people do after adding something to their carts gives Lenovo more confidence around predicting who has a higher propensity to purchase. In turn, it also allows Lenovo to be more efficient with its display spend, basing it on a consumer’s actions, rather than spraying and praying.
A higher level of confidence
After extensive testing, Sivadasan said he is fairly certain this model works and Lenovo is now figuring out how to apply it to its end-to-end journey map.
“There are a few things we need to do to really understand how the model will manifest itself from an experience standpoint and what’s critical to understand to each of the customer sets, and really figure out do we give the same experience for 60 days, 90 days, a year? What’s the protocol?” asks Sivadasan. “My sense is we really want to get it done, so we might test in one country in the next six months.”
After simulations, Lenovo has a high level of confidence that algorithmic retargeting will outperform rules-based retargeting, but the brand needs to implement actual tests now. However, based on initial tests, Braganza said Lenovo appears to be able to drive additional conversions at a lower cost, which means savings for the business overall and more efficiency with how it spends its marketing dollars.
“The beauty of programmatic is the future is going to be about algorithms competing with other algorithms,” he says. “My team is where we want to be with the ability to test the efficacy of different algorithms to optimize our media spend, so it’s ongoing. It won’t be one and done. It’s a start, but, basically, it’s a race to have a better algorithm.”
___
by Jay Marwaha